Object Tracking
We mainly put our emphasis on autonomtive object tracking, especially the extended object tracking.
Tracker uses mainly three elementary knowledge:
noise model: uncertainties.
system dynamics: state evolution and prior information,
measurement system: sensor,
to extract two essential information:
quality, i.e., accuracy and reliability, higher than the raw measurements
inference information, not directly available in the measurements.
For serious Bayesian researchers, it is highly recommended to read [1]:
- Purple Bible:
Estimation with Applications to Tracking and Navigation by Yaakov Bar-Shalom, X. Rong Li and Thiagalingam Kirubarajan.
- Yellow Brick:
Tracking and Data Fusion: A Handbook of Algorithms by Yaakov Bar-Shalom, Peter K. Willett and Xin Tian.
- Grey Manual:
Design and Analysis of Modern Tracking Systems (Artech House Radar Library) by Samuel Blackman and Robert Popoli.
Stone Soup by DSTL
A wonderful point target tutorial for industrial engineers is presented by the Defence Science and Technology Laboratory (DSTL), called Stone Soup.
Stone Soup is developed in Python with six major components: framework, data, algorithms, metrics, simulators, and sensor models. The framework is the core of the project and as a software architecture in a modular fashion integrating all essence in Tracking (such as dynamic/measurement models, noise metrics and simulators).
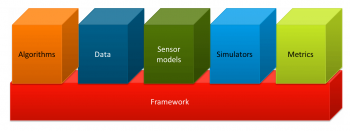
Fig. 01: Stone Soup Structure
One of our major efforts is to design an Extended Object Tracking Framework similar to the Stone Soup.
However, before applying the tracking/estimation theory originated from aerospace systems for automotive applications, we must understand their common ground and differences.
Automotive Tracking versus Aerospace Tracking
Automotive |
Aerospace |
weak Gaussian assumption |
strong Gaussian assumption |
extended object |
point target |
kinematic and shape association |
probablistic association |
Let us call the system OCEAN.
Ocean by UniverSee
The Ocean consists mainly of the following modules:
Framework for extended object tracking (EOT)
Dynamic model set
Measurement model set
Mutltiple model toolbox
Association scheme
Performance metrics
Pre-Processing tool